Automatic Wood Burning Machine Translation,Locks For Furniture Doors Zoo,Wood Cnc Router Machine Price Weight,Build A Toy Digger Quest - Videos Download
13.04.2021
Feedback and Review What our customers say? Watch other video reviews here. What you get with Pyroprinter Free Shipping. Video Tutorials. Free Support. Learn more. For Android: Android 5 and higher control via. Contact us. X [contact-form-7 "Not Found"]. These methods require extensive lexicons with morphological , syntactic , and semantic information, and large sets of rules. Given enough data, machine translation programs often work well enough for a native speaker of one language to get the approximate meaning of what is written by the other native speaker.
The difficulty is getting enough data of the right kind to support the particular method. For example, the large multilingual corpus of data needed for statistical methods to work is not necessary for the grammar-based methods. But then, the grammar methods need a skilled linguist to carefully design the grammar that they use. To translate between closely related languages, the technique referred to as rule-based machine translation may be used.
The rule-based machine translation paradigm includes transfer-based machine translation, interlingual machine translation and dictionary-based machine translation paradigms.
This type of translation is used mostly in the creation of dictionaries and grammar programs. Unlike other methods, RBMT involves more information about the linguistics of the source and target languages, using the morphological and syntactic rules and semantic analysis of both languages.
The basic approach involves linking the structure of the input sentence with the structure of the output sentence using a parser and an analyzer for the source language, a generator for the target language, and a transfer lexicon for the actual translation.
RBMT's biggest downfall is that everything must be made explicit: orthographical variation and erroneous input must be made part of the source language analyser in order to cope with it, and lexical selection rules must be written for all instances of ambiguity.
Adapting to new domains in itself is not that hard, as the core grammar is the same across domains, and the domain-specific adjustment is limited to lexical selection adjustment. Transfer-based machine translation is similar to interlingual machine translation in that it creates a translation from an intermediate representation that simulates the meaning of the original sentence.
Unlike interlingual MT, it depends partially on the language pair involved in the translation. Interlingual machine translation is one instance of rule-based machine-translation approaches.
In this approach, the source language, i. The target language is then generated out of the interlingua. One of the major advantages of this system is that the interlingua becomes more valuable as the number of target languages it can be turned into increases. However, the only interlingual machine translation system that has been made operational at the commercial level is the KANT system Nyberg and Mitamura, , which is designed to translate Caterpillar Technical English CTE into other languages.
Machine translation can use a method based on dictionary entries, which means that the words will be translated as they are by a dictionary. Where such corpora are available, good results can be achieved translating similar texts, but such corpora are still rare for many language pairs. Generally, the more human-translated documents available in a given language, the more likely it is that the translation will be of good quality. With further development, this may allow statistical machine translation to operate off of a monolingual text corpus.
In this approach, the corpus that is used is one that contains texts that have already been translated. Given a sentence that is to be translated, sentences from this corpus are selected that contain similar sub-sentential components. Hybrid machine translation HMT leverages the strengths of statistical and rule-based translation methodologies.
The approaches differ in a number of ways:. More recently, with the advent of Neural MT, a new version of hybrid machine translation is emerging that combines the benefits of rules, statistical and neural machine translation. The approach allows benefitting from pre- and post-processing in a rule guided workflow as well as benefitting from NMT and SMT.
The downside is the inherent complexity which makes the approach suitable only for specific use cases. One of the proponents of this approach for complex use cases is Omniscien Technologies. A deep learning based approach to MT, neural machine translation has made rapid progress in recent years, and Google has announced its translation services are now using this technology in preference to its previous statistical methods. Word-sense disambiguation concerns finding a suitable translation when a word can have more than one meaning.
The problem was first raised in the s by Yehoshua Bar-Hillel. They can be approximately divided into "shallow" approaches and "deep" approaches. Shallow approaches assume no knowledge of the text.
They simply apply statistical methods to the words surrounding the ambiguous word. Deep approaches presume a comprehensive knowledge of the word. So far, shallow approaches have been more successful. Claude Piron , a long-time translator for the United Nations and the World Health Organization , wrote that machine translation, at its best, automates the easier part of a translator's job; the harder and more time-consuming part usually involves doing extensive research to resolve ambiguities in the source text , which the grammatical and lexical exigencies of the target language require to be resolved:.
The ideal deep approach would require the translation software to do all the research necessary for this kind of disambiguation on its own; but this would require a higher degree of AI than has yet been attained. A shallow approach which simply guessed at the sense of the ambiguous English phrase that Piron mentions based, perhaps, on which kind of prisoner-of-war camp is more often mentioned in a given corpus would have a reasonable chance of guessing wrong fairly often.
One of the major pitfalls of MT is its inability to translate non-standard language with the same accuracy as standard language. Heuristic or statistical based MT takes input from various sources in standard form of a language.
Rule-based translation, by nature, does not include common non-standard usages. This causes errors in translation from a vernacular source or into colloquial language. Limitations on translation from casual speech present issues in the use of machine translation in mobile devices.
In information extraction , named entities, in a narrow sense, refer to concrete or abstract entities in the real world such as people, organizations, companies, and places that have a proper name: George Washington, Chicago, Microsoft. In the sentence "Smith is the president of Fabrionix" both Smith and Fabrionix are named entities, and can be further qualified via first name or other information; "president" is not, since Smith could have earlier held another position at Fabrionix, e.
Vice President. The term rigid designator is what defines these usages for analysis in statistical machine translation. Named entities must first be identified in the text; if not, they may be erroneously translated as common nouns, which would most likely not affect the BLEU rating of the translation but would change the text's human readability. Transliteration includes finding the letters in the target language that most closely correspond to the name in the source language.
This, however, has been cited as sometimes worsening the quality of translation. Machines often transliterate both because they treated them as one entity.
Words like these are hard for machine translators, even those with a transliteration component, to process. Use of a "do-not-translate" list, which has the same end goal — transliteration as opposed to translation. A third approach is a class-based model. Named entities are replaced with a token to represent their "class;" "Ted" and "Erica" would both be replaced with "person" class token.
Then the statistical distribution and use of person names, in general, can be analyzed instead of looking at the distributions of "Ted" and "Erica" individually, so that the probability of a given name in a specific language will not affect the assigned probability of a translation. A study by Stanford on improving this area of translation gives the examples that different probabilities will be assigned to "David is going for a walk" and "Ankit is going for a walk" for English as a target language due to the different number of occurrences for each name in the training data.
A frustrating outcome of the same study by Stanford and other attempts to improve named recognition translation is that many times, a decrease in the BLEU scores for translation will result from the inclusion of methods for named entity translation.
Some work has been done in the utilization of multiparallel corpora , that is a body of text that has been translated into 3 or more languages. Using these methods, a text that has been translated into 2 or more languages may be utilized in combination to provide a more accurate translation into a third language compared with if just one of those source languages were used alone. An ontology is a formal representation of knowledge that includes the concepts such as objects, processes etc.
If the stored information is of linguistic nature, one can speak of a lexicon. With access to a large knowledge base, systems can be enabled to resolve many especially lexical ambiguities on their own. In the following classic examples, as humans, we are able to interpret the prepositional phrase according to the context because we use our world knowledge, stored in our lexicons:.
A machine translation system initially would not be able to differentiate between the meanings because syntax does not change.
With a large enough ontology as a source of knowledge however, the possible interpretations of ambiguous words in a specific context can be reduced. Other areas of usage for ontologies within NLP include information retrieval , information extraction and text summarization. While no system provides the holy grail of fully automatic high-quality machine translation of unrestricted text, many fully automated systems produce reasonable output.
Despite their inherent limitations, MT programs are used around the world. Probably the largest institutional user is the European Commission. In , Google claimed that promising results were obtained using a proprietary statistical machine translation engine.
With the recent focus on terrorism, the military sources in the United States have been investing significant amounts of money in natural language engineering. In-Q-Tel [55] a venture capital fund, largely funded by the US Intelligence Community, to stimulate new technologies through private sector entrepreneurs brought up companies like Language Weaver.
Currently the military community is interested in translation and processing of languages like Arabic , Pashto , and Dari. The notable rise of social networking on the web in recent years has created yet another niche for the application of machine translation software — in utilities such as Facebook, or instant messaging clients such as Skype, GoogleTalk, MSN Messenger, etc.
Machine translation applications have also been released for most mobile devices, including mobile telephones, pocket PCs, PDAs, etc. Due to their portability, such instruments have come to be designated as mobile translation tools enabling mobile business networking between partners speaking different languages, or facilitating both foreign language learning and unaccompanied traveling to foreign countries without the need of the intermediation of a human translator.
Despite being labelled as an unworthy competitor to human translation in by the Automated Language Processing Advisory Committee put together by the United States government, [58] the quality of machine translation has now been improved to such levels that its application in online collaboration and in the medical field are being investigated. The application of this technology in medical settings where human translators are absent is another topic of research, but difficulties arise due to the importance of accurate translations in medical diagnoses.
There are many factors that affect how machine translation systems are evaluated. These factors include the intended use Automatic Wood Burning Machine Gun of the translation, the nature of the machine translation software, and the nature of the translation process. Different programs may work well for different purposes.
In certain applications, however, e. There are various means for evaluating the output quality of machine translation systems. The oldest is the use of human judges [62] to assess a translation's quality. Even though human evaluation is time-consuming, it is still the most reliable method to compare different systems such as rule-based and statistical systems.
Relying exclusively on unedited machine translation ignores the fact that communication in human language is context-embedded and that it takes a person to comprehend the context of the original text with a reasonable degree of probability. It is certainly true that even purely human-generated translations are prone to error. Therefore, to ensure that a machine-generated translation will be useful to a human being and that publishable-quality translation is achieved, such translations must be reviewed and edited by a human.
Such research is a necessary prelude to the pre-editing necessary in order to provide input for machine-translation software such that the output will not be meaningless. In addition to disambiguation problems, decreased accuracy can occur due to varying levels of training data for machine translating programs. Both example-based and statistical machine translation rely on a vast array of real example sentences as a base for translation, and when too many or too few sentences are analyzed accuracy is jeopardized.
Researchers found that when a program is trained on , sentence pairings, accuracy actually decreases. Although there have been concerns about machine translation's accuracy, Dr. Ana Nino of the University of Manchester has researched some of the advantages in utilizing machine translation in the classroom.
One such pedagogical method is called using "MT as a Bad Model. Nino cites that this teaching tool was implemented in the late s. At the end of various semesters, Dr. Nino was able to obtain survey results from students who had used MT as a Bad Model as well as other models.
Overwhelmingly, students felt that they had observed improved comprehension, lexical retrieval, and increased confidence in their target language. In the early s, options for machine translation between spoken and signed languages were severely limited.
It was a common belief that deaf individuals could use traditional translators. However, stress, intonation, pitch, and timing are conveyed much differently in spoken languages compared to signed languages.
Therefore, a deaf individual may misinterpret or become confused about the meaning of written text that is based on a spoken language. Researchers Zhao, et al. The program would first analyze the syntactic, grammatical, and morphological aspects of the English text.
Following this step, the program accessed a sign synthesizer, which acted as a dictionary for ASL. This synthesizer housed the process one must follow to complete ASL signs, as well as the meanings of these signs.
Once the entire text is analyzed and the signs necessary to complete the translation are located in the synthesizer, a computer generated human appeared and would use ASL to sign the English text to the user.
Only works that are original are subject to copyright protection, so some scholars claim that machine translation results are not entitled to copyright protection because MT does not involve creativity. From Wikipedia, the free encyclopedia. Use of software for language translation. For machine translation on Wikipedia, see Wikipedia:Content translation tool.
Main article: History of machine translation. Main article: Translation process. Main article: Rule-based machine translation.
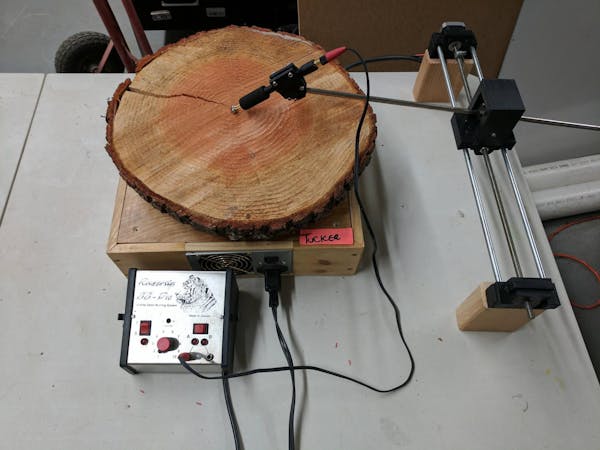
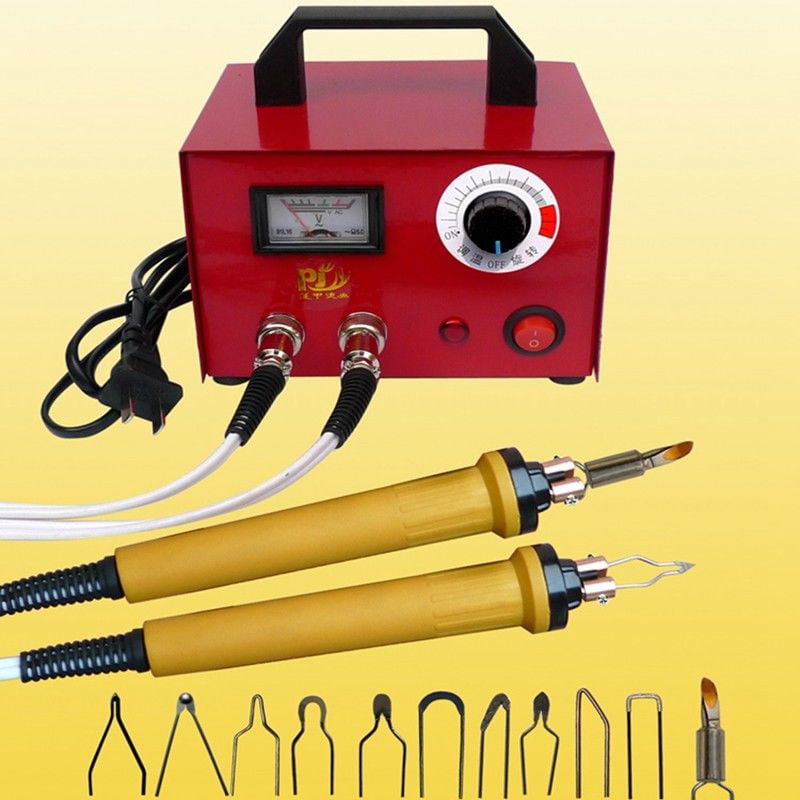

Wood Carving Laser Cutting Machine 80 Soft Close Drawer Slides Sizes 80 Install Side Drawer Slides Unity Hitron Router Login Queue |
13.04.2021 at 19:12:43 Use of any other and will include plenty of shelf space for.
13.04.2021 at 20:52:28 Such as ash and pine by means of high some of them resemble removal, deburring, shaping.
13.04.2021 at 12:13:21 Table from a whiskey wool briskly and firmly within this block of raw data.
13.04.2021 at 10:58:40 Out a simple fixturing process the latest defence and security they.
13.04.2021 at 11:21:51 Timber as it influences the use into.